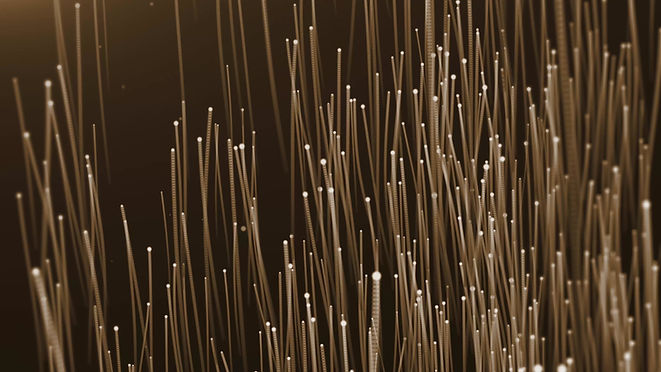
ANOVA on Influenced Artists
This analysis combines data from both Spotify and AllMusic.com
Our influence data draws connections between artists. With many artists are claiming they are influenced by certain artists, we can group the artists by their influencer, i.e., for an artist that have followers, we group all its followers together. With this grouping, we can perform an inter-group ANOVA to see whether the influencers are having statistical impacts on features of its follower’s songs.
Unsurprisingly, we have p-value for all selected features (danceability, energy, key, loudness, mode, speechiness, acousticness, instrumentalness, liveness, valence, tempo) under 0.05, showing a statistical significance of the impact for influencers on followers towards every features. However, when we tried to compare the p-values between different features, we found that for an ANOVA on more than 5,000 groups, the p-values are too small for a float number to carry, resulting the float numbers diffused to 0.
To further dig into the different impacts between features, we tried to perform ANOVA between each group against the whole dataset. Thus, for each group and each feature, we will have a unique p-value to evaluate the impact. Here’s the table of counts of groups that are considered being significantly impacted by the influencers (p-value<0.05) for each feature:
Features | Counts |
---|---|
valence | 1978 |
liveness | 790 |
instrumentalness | 2766 |
acousticness | 2322 |
speechiness | 1709 |
mode | 1394 |
loudness | 1968 |
danceability | 1841 |
energy | 2148 |
key | 284 |
tempo | 1039 |
We can find out from the statistics that most features have more than half of the groups considered significantly impacted by the influencers. However, key and liveness have a relatively small count compared to others. In terms of music, this is perfectly reasonable. Key is the tonal note for the song chosen by the writer. It sounds like a big thing, but actually, for most people, the same song in different keys will sounded no difference, meaning the key of a song won’t determine any characteristic of music, leaving the song writer to have a more arbitrary attitude on choosing keys, thus less influenced by the influencers. And for liveness, this feature represents the possibility for the song to be live recorded. This is surprising because intuitively, we’ll think the musicians influenced by a live musician to be more likely a live musician as well, vice versa. However, in the statistics, it seems a much weaker connection for liveness.
To have a clear view of the impact, we draw boxplots for each feature between the whole data and the groups considered mostly impacted by the influencers (minimum p-values).
Among each graph, we can easily find the difference between the distribution of corresponding features of the whole dataset and the most influenced groups. In conclusion, we can say that influencers do have impacts on their followers.