
Conclusions & Future
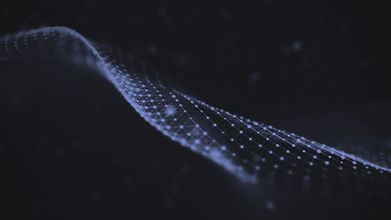
Limitation
Clustering models randomly sample data, so the results may have minus differences.
The classification models are based on clustering groups, maybe there has been a more appropriate cluster model for songs. Also, the genres are unbalanced so it causes the bias when exploring the features of different songs.
There are a lot of missing data about influence in text data. In addition, although we tried using topic models to analyse text data, as the subjects of these data are overly direct regarding about introduction and influence, the results are meaningless.
We could only subplot the influential directed graph instead of completely exhibition due to its large size.
Classical music has been influencing others for the longest time, while other genres may update faster. Through Anova, we prove that followers are do influenced by their influencers.
The musicians around the artist who has higher influence has less possibility to influence others. Otherwise, in a small particular genre, the musicians are more possible to influence each other. Also, we find the trend that as the time goes, the music becomes louder.
For the songs, loudness and energy are the most correlated features. Different songs has their unique characteristics but we can't use a traditional classify method to categorize them well.
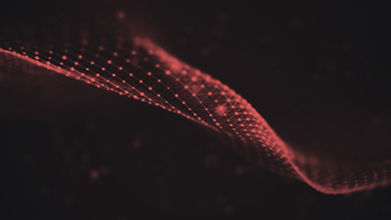
Conclusions
We all collect data from public data sets and accessible APIs so we don't have the issue of lack of transparency and violating privacy.
However, we don't collect data from different sources only from the main musical apps, which would cause bias and omit some information.
(i.e. The older may not use these apps)
The distribution of our data are unbalanced, most of them gather in the range from 1980 to 2020. Hence, there aren't sufficient early data resulting in the bias between songs from different generation.
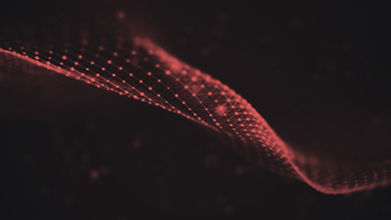
Ethical considerations
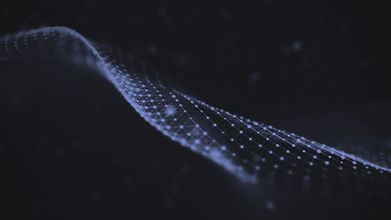
Future
Collect comprehensive data to get rid of bias and collect data from different resources like magazines and newspaper.
Explore mutual influence within genres than only musicians.
Use text clustering and Ner skill to extract the influential flows from the text data.
Exploit high-level graph algorithms to find more useful information in the influential graph.