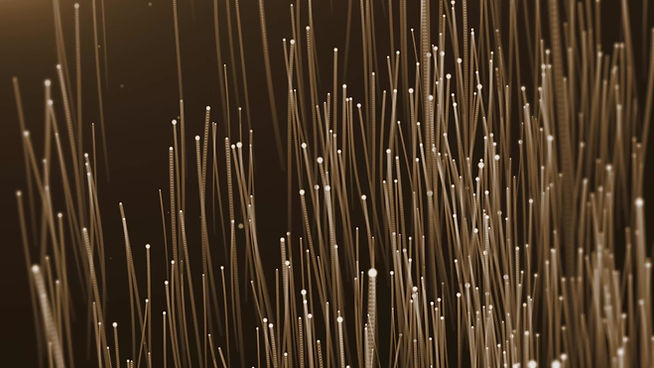
Statistical analysis
Basic statistical value of features and anomaly detection about them.
Basic statistical analysis
We choose the attribute in songs datasets. Attributes are :
‘danceability’, ’energy’, ’key’, ’loudness’, ’mode’, ’speechiness’, ’acousticness’, ’instrumentalness’, ’liveness’, ’valence’, ’tempo’.
These attributes represent the feature of the songs, which also show the degree in different features. Most of them are limit from 0 to 1, except the key, loudness, and tempo. The attribute of key, loudness, and tempo are the real unit in reality. The range of these 3 attribute are the key from 0 to 11, the loudness smaller than 0 and the tempo more than 0.
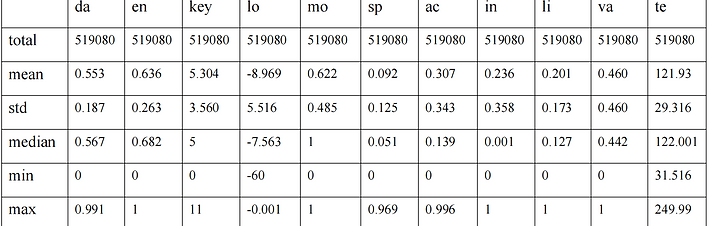
In the influence data, the mean of the influence gap year which we add is 15. It means that most musicians are influenced by those in the former generation. Also, the mode of genres is pop/rock. We think it corresponds with the intuition that most musicians are involved in these two areas.
Anomaly detection
We use the local outlier factor (LOF) to get the anomaly in song's attribute. There were 3 k being used, which are 10, 20, 30 and the contamination are set to be 0.001.

By checking the ‘anomaly’ data, it is showing that the ‘anomaly’ data has at least one attribute which are very close to the maximum or minimum. But considering the music diversity, these ‘anomaly’ data of songs can make sense, because there are many experimental music or innovative music being produced these day, which are far beyond people’s imagination. Besides, these data are not out of bounds. It is still in the human understanding. What is more, we randomly search some of the song with anomaly’ data, and found out that these songs are really exist. Therefore, we believe the 'anomaly' data are not data issue, so we design to keep these data.